EMo2Cla aims to explore how to adapt and improve the teaching/learning processes that take place physically in the classroom through affective computing using physiological and inertial sensors. The key issue here is combining the affective computing with the movements students make during the learning activity.
While this can be useful in diverse educational situations (such as to analyze the voluntary gestural movements of the students during their interaction with the teacher), in a collaboration with Dr. Martinez Maldonado we have focused on exploring stress reactions in a classroom teamwork work scenario in the context of healthcare (specifically, nursing), where teachers commonly have a series of expectations about the moments in which students will have a higher cognitive load and stress that can impact on their learning, depending on the phase of the educational training in which the students are and how they move around the space prepared for the training.
In particular, the research work carried out to the moment explored how to process the multimodal data obtained in a dataset with 50 participants which was collected with the Empatica e4 physiological wristband, a medical-grade wearable device that offers real-time physiological data acquisition including electrodermal activity, wrist acceleration and body temperature. The multimodal data processing performed focused on identifying if the arousal levels captured during the simulation based learning scenario match the teachers’ expectations regarding the students’ reactions to stressful situations in each of the predefined phases of the learning activity, which consisted in five different phases with a critical patient, and in which students must learn to make life-to-death decisions timely.
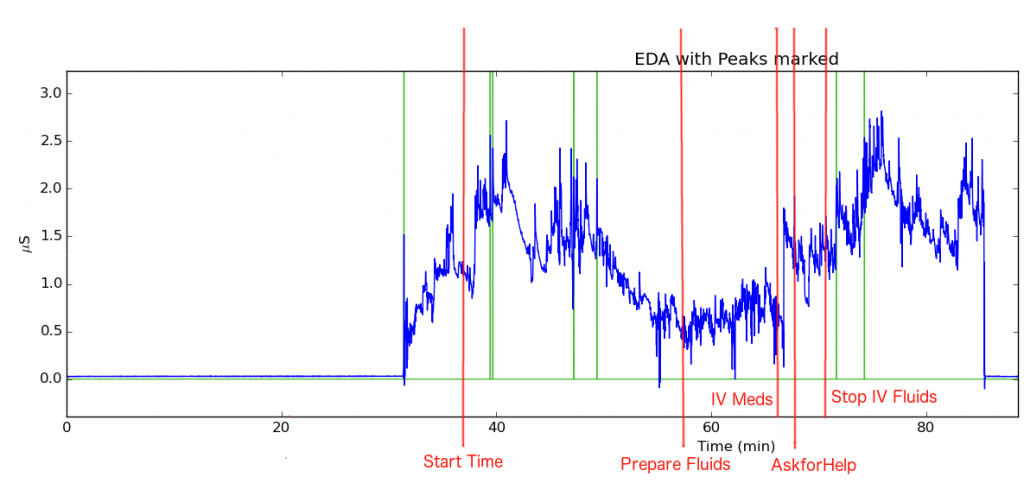
Thus, this research focuses on evaluating and enriching the performance of learning teams in group tasks that are developed in physical environments, making the traces of the movements performed visible to facilitate computational analysis. The work carried out so far suggests investigating the combination of physical aspects of the students’ behavior during the learning activity (in this case, in relation to positioning and movements through the physical space of the room) with their affective state. In this way, proximity, motion and location analytics are combined with affective computing in a team-based healthcare simulation classroom.
Selected References:
M. A. Ronda-Carracao, O. C. Santos, G. Fernandez-Nieto, & R. Martinez-Maldonado (2021), “Towards Exploring Stress Reactions in Teamwork using Multimodal Physiological Data”, 1st International Workshop on Multimodal Artificial Intelligence in Education, MAIED 2021.
R. Martinez-Maldonado, V. Echeverria, O. C. Santos, A. D. P. D. Santos, & K. Yacef (2018), “Physical learning analytics: A multimodal perspective”, en Proceedings of the 8th International Conference on Learning Analytics and Knowledge.
R. Martinez-Maldonado, K. Yacef, A. Dias Pereira Dos Santos, S. Buckingham Shum, V. Echeverria, O. C. Santos & M. Pechenizkiy (2017), “Towards proximity tracking and sensemaking for supporting teamwork and learning”, in 2017 IEEE 17th International Conference on Advanced Learning Technologies (ICALT).